How does artificial intelligence figure out human language to create its own learning curve? Christian Keszthelyi talks to one of Malta’s leading AI researchers, Dr Claudia Borg.
Machine learning today means that, if given appropriate architecture, a machine can absorb and store information by itself. Amid the buzz around artificial intelligence (AI), Dr Claudia Borg, a Maltese morphology (word formation) expert, decided to find ways for utilising AI for language processing — a subfield focusing on programming computers to analyse natural language data. It led her to the contested topic of how users’ bias affects software development.
Borg is happy to shatter the image of AI researchers as IT-obsessed prodigies. She tells me about being considered a slow learner as a child, late in learning to read and write, receiving bad marks, and failing exams in several subjects. Her school results started improving at the age of 12, with extracurricular help. She did not consider herself a high-achiever at that age, and struggled to discover what motivates her after graduation. ‘I would jump from job to job because I found them boring. For example, I have done the accounts of a company for this year, the next year I will do the same. There is not going to be anything new or more challenges, so, what’s next?’ she recalls. Borg gave a programming course a try and fell in love, finally discovering ‘something very logical, step by step,’ that lead to an aha-moment. Being posed with a problem and seeking solutions appealed to her.
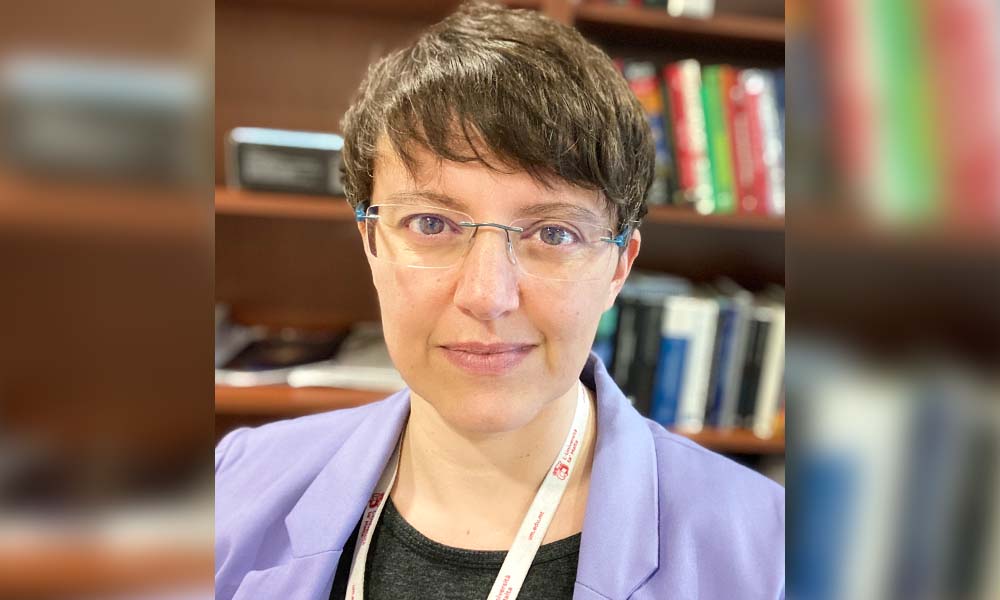
Having entered the University of Malta (UM) as a mature student, Borg got involved in a research project on natural language processing for her Master’s degree. She worked with software to parse pieces of text, identify the keywords, and give definitions to assist e-learning. She started working with the Maltese language for her research, and her curiosity and love for the logical led her to complete a PhD, looking at ways to break down Maltese words to understand the grammatical meaning behind the chunks. Fast forward some years and you will find her as a lecturer in Artificial Intelligence — among many other subjects — at the Faculty of Information & Communication Technology (UM).
A computer brain?
Borg walks me through the development of the logical, algorithmic world of AI. Today’s machine learning uses neural networks. As a technique, it has been around since the 1980s, though to gain popularity, it needed to wait until increases in machine power allowed researchers to build bigger networks that were a lot more powerful. ‘Neural networks work just like the brain. There are cells of information that are connected, and only certain cells will fire up when information is activated, based on what is needed or what is happening,’ Borg elaborates. ‘Researchers set up the architecture of the network of these neurons, but the learning happens on its own.’
Every cell in a computer-based neural network is a mathematical function. When a signal comes into a cell, it will either send a signal to the next cell it is connected to, or remain dormant. ‘We no longer provide specific linguistic features. We have algorithms that can learn these features on their own,’ Borg says. Neural networks are like black boxes — researchers do not really know what happens in them unless they look inside and analyse them. ‘We open this box and hook up a different algorithm to certain aspects to see what is going on,’ Borg says. This area is called explainable AI.
Borg gave a programming course a try and fell in love, finally discovering ‘something very logical, step by step,’ that lead to an aha-moment. Being posed with a problem and seeking solutions appealed to her.
Before, with statistical techniques, the machine learning process was very transparent, but today it takes quite a while for researchers to understand how the network learns, since researchers do not input the features anymore. With statistical techniques the process was much faster — researchers would mock up the model and it would work. Deep learning requires much more data than traditional statistical machine learning methods.
Yet this is what makes AI more human-like. It takes more than a year before toddlers start uttering words. In all that period the baby keeps absorbing data. Likewise, deep learning takes its time depending on the architecture and the processing power of the machine. This is why big tech companies keep collecting data on the go. Borg hopes that one day corporations will share their data with academics for research.
Borg’s field has a problem: data for the Maltese language are scarce. To overcome the problem, researchers need to think outside the box. Transferring the knowledge they have from high-resource languages such as Italian, Spanish, and English, they can do cutting-edge research related to Maltese. Since Maltese is influenced by multiple languages, researchers can input features — language characteristics — from these languages. This allows the machine to process more training data, bypassing the problem of the lack of data in Maltese. Trained with additional data, AI tools can then study Maltese sentence structure, grammatical analysis, and machine translation.
Most AI we see in movies and popular media, outwitting humans, are not possible today. Today’s AI is task-specific and narrow. To work well, it needs a specific focus on a task or domain. ‘The aim of General AI is to have a singular machine that is able to learn and communicate about any subject in an autonomous manner. So far, we do not have the evidence that we will reach this milestone any time soon. However, we do need to think about the implications that such a system would have on every aspect of our lives, and we need to ensure that we have a strong ethical framework in place,’ Borg says.
Human bias; Machine bias
In 2016, Microsoft launched an AI bot on Twitter, Tay, that absorbed knowledge from tweets. Quickly the ‘machine’ started sympathising with Hitler’s ideas, denying the Holocaust, and using racial and sexual slurs. Microsoft gave limitless access to Tay, and forgot to factor in that people can be awful. But how can we prevent AI from learning the socially unacceptable? One of the problems with AI is that we, as people, have bias – conscious or not, Borg explains. ‘[Let’s say,] I walk into my classroom and ask everybody to draw a pair of shoes, the first shoe that comes to their mind. Because I have a gender bias — not a lot of women in my class — I am going to get sneakers and not high heel shoes.’
Today’s AI is task-specific and narrow. To work well, it needs a specific focus on a task or domain.
So how can we overcome our individual biases affecting the solutions we create? ‘What we need to do is to educate ourselves better and become more aware of our own inherent biases. Diversity is key. Our biases pose challenges to AI […] We are aware of our biases, and still they keep on happening,’ Borg reflects. These distortions affect the usability of AI tools.
Natural language processing is one of the perfect examples where such biases can be explored. Machine translation might automatically translate a sentence using a male pronoun because it is more present in the training data, which contains the hidden biases of developers. Researchers around the world are trying to see how to remove such biases and balance things out.
‘We need to take a step back and focus on education. When creating technology, we must be aware of our biases, conscious or unconscious. Which is why it is so important to have diversity within our workforce. It is not a matter of gender balance or any other quota. It is simply a matter that if something is to serve everyone, then everyone needs to be represented. Only then can we ensure that our biases do not end up in the systems we develop,’ Borg says.
This article was written in collaboration with Business Malta.
Comments are closed for this article!