As passengers, we often overlook the complexity and challenges faced by pilots as they navigate the skies. THINK explores SmartAP, a cutting-edge AI technology that could help pilots combat stalling and difficult landing situations. Buckle up, sit back, and enjoy the journey!
On-board automation, such as autopilot and the Flight Management System, plays a central role in operating a modern airliner and reduces pilot workload significantly. Yet, even with these aids, flying a plane is still far more challenging than driving a manual! Despite the advanced technology and rigorous training undertaken by pilots, fatal accidents can still occur, especially if the onboard automation and protection systems fail. For instance, the aircraft may enter a stall, where the aircraft is unable to generate lift and starts losing altitude. This may lead to a crash unless corrective action is taken. In another scenario, the pilots might struggle to visually identify the destination airport and runway from the air – due to adverse weather conditions, nighttime, or unfamiliarity with the airport – as they approach their destination. This could risk an unsafe landing.
A group of researchers at UM, in collaboration with QuAero Ltd., have developed an artificial intelligence (AI) system to help pilots in these specific scenarios. While there are already various on-board systems, SmartAP is designed to augment these systems should they fail, acting as an extra pair of eyes on the flight deck.
SmartAP: AI for Pilots in Critical Scenarios
‘The application of AI in aviation is still in its infancy,’ explains Dr Ing. Jason Gauci (Senior Lecturer at the Institute of Aerospace Technologies). ‘There aren’t yet any certified AI-enabled systems for use on large transport aircraft, and so far, AI is being used for non safety-critical applications.’ However, the European Union Aviation Safety Agency (EASA) – as well as other major aviation stakeholders – has recognised the importance of AI and developed a roadmap outlining the integration of AI into the aviation industry.
SmartAP focuses on two specific scenarios: recovering from stalls and identifying airports. While it could help pilots recover the aircraft in potentially dangerous scenarios, it could also facilitate the final phases of flight by highlighting airports and runways in the external view (i.e. outside the cockpit) through different weather conditions and times of day. To develop the AI, the researchers used training data as well as different machine learning techniques. Let’s take a closer look at how they trained the AI in both scenarios.
Training the AI: Stalling and Airport Identification
The aircraft’s wings are angled towards incoming air to generate lift. This angle is called ‘the angle of attack’. Stalling occurs when the angle of attack exceeds a value called the ‘critical angle of attack’, causing a disruption in the airflow over the wings. When an aircraft stalls, it loses its ability to generate sufficient lift, leading to a decrease in altitude as gravity becomes the dominant force.
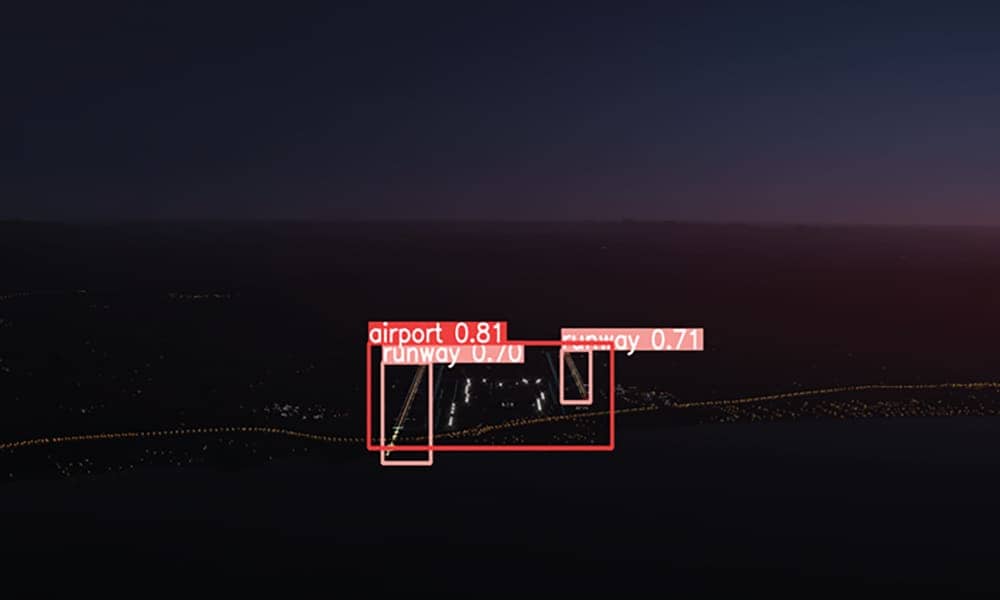
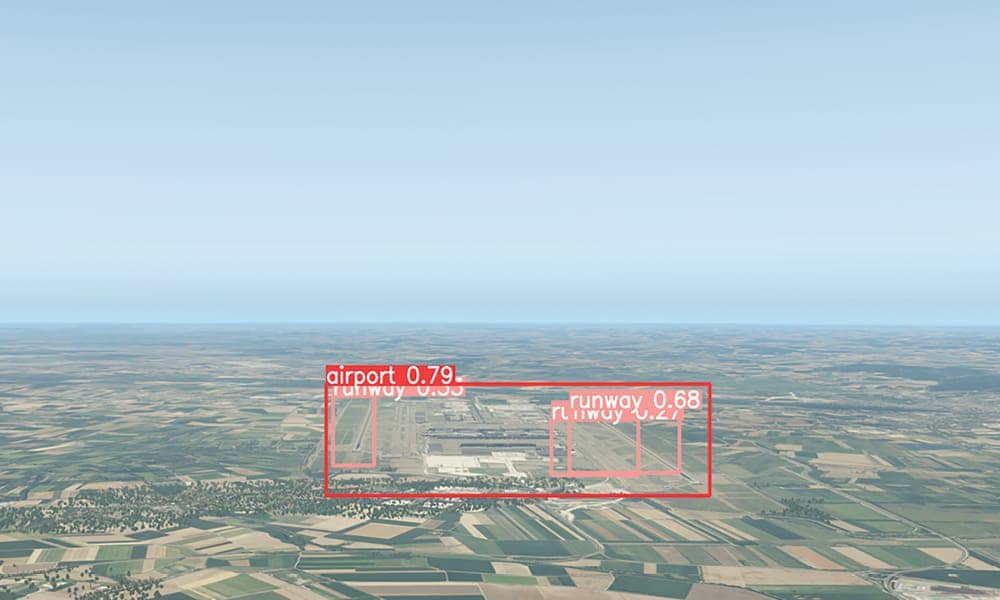
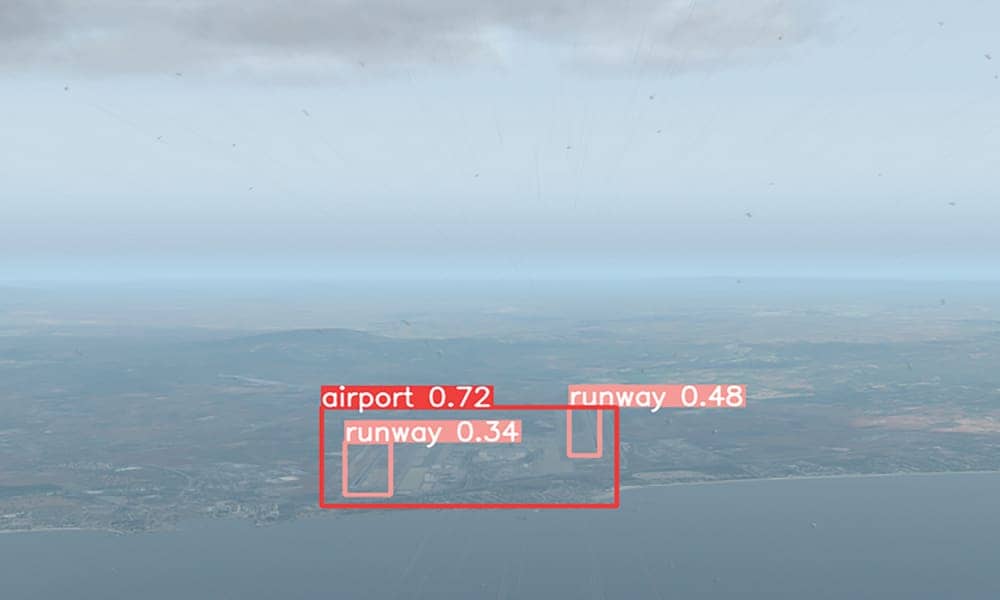
Results obtained when testing the AI with a number of images of airports and runways at various distances and weather conditions. The numbers are the confidence values of the algorithm. Images courtesy of Dr Ing. Jason Gauci
To teach the AI to recover from a stall, the researchers used reinforcement learning. Reinforcement learning allows an AI to learn on its own through a process of trial and error. The researchers define a ‘reward function’, and the AI takes different actions, receives feedback from the aircraft, and adjusts its behaviour based on the rewards – gradually refining its decision-making process to maximise the rewards it receives. Gauci says ‘The rewards depend on multiple factors, such as the time it takes the AI to recover the aircraft from a stall and the height that is lost during the recovery process. The quicker the recovery and the smaller the height loss, the higher the reward given.’
Training the AI takes a lot of time. In order to speed up the training, the researchers pre-trained the AI using a technique called behaviour cloning. ‘We had an experienced commercial airline pilot (from QuAero Ltd.) come in to perform stall recoveries in a variety of conditions using flight simulation software. We recorded all of that data and used it to pre-train the AI.’
Adverse weather conditions – such as heavy rain or fog – and nighttime can significantly reduce a pilot’s visibility and make it challenging to visually identify landmarks such as airports and runways. ‘To identify airports and runways, we used a different type of machine learning, specifically a pre-existing object detection algorithm called “YOLO” (You Only Look Once).’ YOLO can be used to detect different types of objects such as people, cars, and fruit. The researchers adapted it to recognise generic airports and runways and fed the algorithm numerous real and synthetic training images of airports and runways taken from different angles, at different heights, and in various weather conditions. These images were all labelled to help train the AI. ‘The idea is to get a big and diverse data set. We put bounding boxes around each airport and runway in each image, essentially telling the AI, “look, this is an airport or runway.”’ The researchers then used a different set of images to test the AI. ‘The AI detects if there is a runway in the image, bounds it in a box, and provides a confidence value – i.e. how confident the AI is that it is correct.’
Unsurprisingly, one of the biggest challenges for the researchers was training the AI itself. ‘In the case of stall recovery, we had to record many stall scenarios with a human pilot, and we had to conduct a large amount of tests. On the other hand, for airport and runway identification, we needed to use a large amount of images, all of which needed to be manually tagged.’ Manually tagging images is a labour-intensive process due to the sheer amount of data. The project took three and a half years. ‘It was an iterative process; a lot of the time was spent labelling the data, training the algorithms, and tweaking them.’
Gauci points out that, ‘we have only scratched the surface with AI in aviation. We didn’t have time to train it to recover from every type of stall, for example. In our case, we focused primarily on stall scenarios which happen when the aircraft is straight and level (also known as 1G stalls) and during a turn (when the aircraft is banked).’
AI and Aviation: The Controversial Future
While a number of intricate systems already exist to help pilots, ‘there can be cases where the automation fails,’ explains Gauci. Air France Flight 447, which crashed in the Atlantic Ocean on its way from Brazil to France, entered a stall condition on 1 June 2009. ‘In this case, the automation systems failed, and the last line of defence was the pilots. Unfortunately, the pilots themselves did not realise that the aircraft was stalled and did not take appropriate corrective action. In this case, AI systems can assist and help prevent these tragedies.’
The question remains though, whether one day we will be flown around exclusively by AI. Gauci is quick to point out that, ‘this is a very controversial topic in the aviation industry.’ Major aircraft manufacturers, such as Airbus and Boeing, are pushing for reduced-crew operations and single-pilot operations to address the shortage of pilots. Currently, passenger-carrying aircraft operate with two pilots (three for transatlantic flights). While historically there were more pilots, this number gradually reduced as the extent of automation increased.
With a single-pilot operation, you’d have one pilot in the air and a ground-based pilot who would be monitoring multiple aircraft or assisting the airborne pilot in the event of an emergency situation. Pilots believe this would reduce safety significantly, while public acceptance is an issue as well. ‘We’d certainly need to have more sophisticated automation systems,’ points out Gauci. According to the EASA Roadmap, ‘the ultimate step towards autonomy [autonomous AI] is most probably to be shifted even after 2050, considering the current state of the art in AI technologies.’
Until then, AI such as SmartAP will improve safety operations. SmartAP is an additional tool to assist pilots in certain challenging scenarios. In the case where an aircraft is coming in to land, it can serve as another pair of eyes, helping pilots identify the airport, or it could help pilots recover from a dangerous stall. However, Gauci makes it clear, ‘it is there to augment, not replace. The pilots still have the final say, essentially.’
The work described in this article was carried out as part of the SMARTAP (R&I-2018-010-T) project which was financed by the Malta Council for Science & Technology, for and on behalf of the Foundation for Science and Technology, through the FUSION: R&I Technology Development Programme.
Author
-
Before taking over as editor of THINK Magazine, David used to teach English, British Literature, and History. He has worked as a freelance writer for a number of companies and has written about a variety of topics; from mattress reviews to cryptocurrency to marketing guides. When he isn’t co-ordinating THINK’s strategy, David enjoys drinking black coffee and plotting global domination.
Comments are closed for this article!