In his book This is Your Brain on Music, Daniel Levitin writes, ‘Whenever humans come together for any reason, music is there.’ From weddings and funerals, to graduation parties and men marching off to war, ‘music is and was [always] part of the fabric of everyday life.’
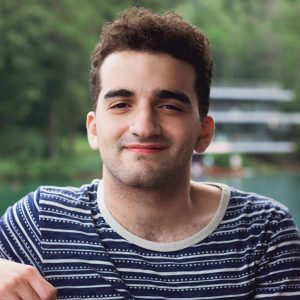
Music is an essential part of human culture, and preservation is key. Thanks to computers, we can digitally store music, and this does not just mean preserving recordings: Optical music recognition (OMR) refers to a discipline that investigates how scanners or cameras can automatically transform scanned sheet music into a computer-readable format.
There are many applications for OMR. For one, a musician can listen to a piece of music before trying to learn it, by converting the printed score sheet into an audio file. Beginners, on the other hand, can play along to the music. OMR could also be performed on a musical score for re-configuration of the music by, for example, transposing it to a more suitable key, or re-writing it for musicians with special reading requirements, like Braille for the visually impaired.
Research in OMR started in the 1960s when scientist Dennis Pruslin first attempted the automatic recognition of sheet music. From there, the field of OMR flourished, seeing the creation of systems such as the MUSER, LEMON, and CANTOR. All these systems achieved high performance values above 95% accuracy.
However, these systems had their restrictions. Only simple score sheets could be read. Most systems ignored basic musical rules such as accidentals (symbols placed before a note on a music sheet in order to raise or lower the pitch of a note). Building on this, the goal of Joseph Bezzina’s research (supervised by Dr Alexandra Bonnici) was to create an OMR system capable of reading complex score sheets acquired using a mobile or tablet camera, instead of a scanner.
To implement the OMR system, Bezzina loosely followed the structure most researchers have used. The structure is made up of three sub-tasks, namely: staff line (the long horizontal lines in a music sheet) identification and isolation, symbol localisation, and musical recognition. In the staff line detection and isolation stage, staff lines are removed from the score sheet image, since staff lines make the next step harder. In the symbol localisation stage, each different symbol is localised and grouped with similar symbols. In the final stage, the pitch of each individual note is measured and musical rules are implemented to give the different symbols a musical meaning. All this data is then converted to MIDI format in order for the musical piece to be able to be heard digitally.
With this system, a precision rate of 94% was achieved when tested with complex musical scores containing accidentals and key signatures which affect the note’s pitch value. In the future, Bezzina and his team wish to extend this work to include a larger variety of music notation, especially notation associated with music’s expressive quality.
Comments are closed for this article!