Have you ever wondered how big or old the universe is? Actually, scratch that; how would you even measure it? A group of scientists at UM hope to answer these questions through machine learning.
The Hubble Constant Crisis is a major conundrum in the field of cosmology. It refers to a difference in the measurement of how fast the universe is expanding. This parameter is fundamental in determining the age, size, and ultimate fate of the universe.
Currently, two main methods are applied to measure the Hubble Constant. One is by observing the cosmic microwave background radiation that was left over from the Big Bang, as well as other measurements from the early Universe such as its chemical composition. The other is by observing the distances and velocities of nearby galaxies. However, the end results of these two methods differ, with a considerable gap between them. The value of the Hubble Constant derived from the cosmic microwave background is around 67.4 kilometres per second per megaparsec (km/s/Mpc), while the value derived from observations of nearby galaxies is around 73.3 km/s/Mpc. Either one of these results is false, or both of them are. This difference in measurements is the heart of the Hubble Constant Crisis.
To understand how the universe expands, we need to speak about how it was formed. ‘We do not fully understand what happened at the earliest times that led to the Big Bang,’ Prof. Jackson Said, Associate Professor at the Institute of Space Sciences and Astronomy, tells THINK. ‘But we do understand what happened just after the Big Bang, thanks to particle physics. We have a good idea of how matter came into being.’
Around 380,000 years after the Big Bang, the explosion cooled enough to make the universe transparent. Then the universe went through what scientists call the Dark Ages, when no stars studded the intergalactic sky. Once stars appeared, the first galaxies started forming while the universe kept expanding. The background radiation that scientists measure is the residue from the Big Bang – similar to how smoke from fireworks gradually dissipates, except on a cosmic scale. While the smoke from fireworks usually vanishes after a minute or two, the background radiation from the Big Bang takes millennia.
The second method relies on measuring the distance and velocity of nearby galaxies. It assumes that the universe is expanding at a constant rate (known as Hubble’s Constant). By knowing the speed and distance of other galaxies, scientists are able to calculate how long it took the galaxies to reach their current position – or, in other words, the age of the universe. Just like we can calculate speed by dividing distance over time, we can calculate time by dividing distance over speed. Of course, it is hardly that simple!
One Small Step for Man…
The Cosmoverse COST Action is an interdisciplinary research network that is aimed at advancing our understanding of the fundamental physics of the universe. The initiative is supported by the European Cooperation in Science and Technology (COST) and is focused on exploring new approaches and techniques for studying the cosmos, from the smallest subatomic particles to the largest-scale structures in the universe.
One of the significant challenges that the network is seeking to address is the Hubble Constant Crisis. By fostering collaboration and knowledge-sharing across different fields, the Cosmoverse network aims to contribute to resolving the Hubble Constant Crisis, advancing our understanding of the universe’s evolution.
Using novel techniques of machine learning as a replacement for traditional methods, they can decrease the noise that naturally appears in observational data, leading to clearer data.
This is where Said’s project, IntelliVerse: Intelligent Precision Cosmology in the Era of New Physics, comes into the picture with machine learning and artificial intelligence. Funded by the Malta Digital Innovation Authority under the MDIA AI Applied Research Grant (MAARG), the project aims to incorporate what has already been developed in this area with the power of AI.
Using novel techniques of machine learning as a replacement for traditional methods, they can decrease the noise that naturally appears in observational data, leading to clearer data. An example of this is seen in observations of the Hubble parameter, which is a measure of the expansion velocity of the universe. This expansion varies in time across the history of the universe, and understanding this profile is important for understanding the underlying physics that govern the dynamics of the universe.
‘We use historical data and run it through a neural network to get a model of the universe’s expansion,’ Dr Jurgen Mifsud, the lead researcher on the project says. ‘We use machine learning to analyse big data that would otherwise be too long for humans to vet. Additionally, humans have psychological predispositions, but machine learning does not,’ Mifsud adds. While not even machine learning can be 100% objective, experience shows that this approach takes away a lot of the known biases we have.
Using machine learning approaches to rebuild the expansion profile of the universe means that the researchers are not assuming any underlying physical model, which is a powerful result as they can then use that profile to fit different models of cosmology. In a way, they are using the data to pick the best model that works rather than the traditional approach of picking a model and using the data to fit its parameters. ‘We have also applied this approach to other cosmological parameters such as those related to the evolution of the large-scale structure of the universe, which explains why we see the number of galaxies that we see in the universe,’ says Said. The project is unique as the researchers have a lot of experience doing both foundational physics and theoretical physics. Coupled with machine learning to handle the data analysis, the outcome of the research is ambitious. But there are many challenges.
‘We have to find the proper mechanism that will be applicable to our data. We are testing neural networks, which takes a lot of time. We have to organise a test, and run the analysis on one of the clusters at the university, which uses a lot of resources. We have to factor in the limitations of the available computational power,’ Said says.
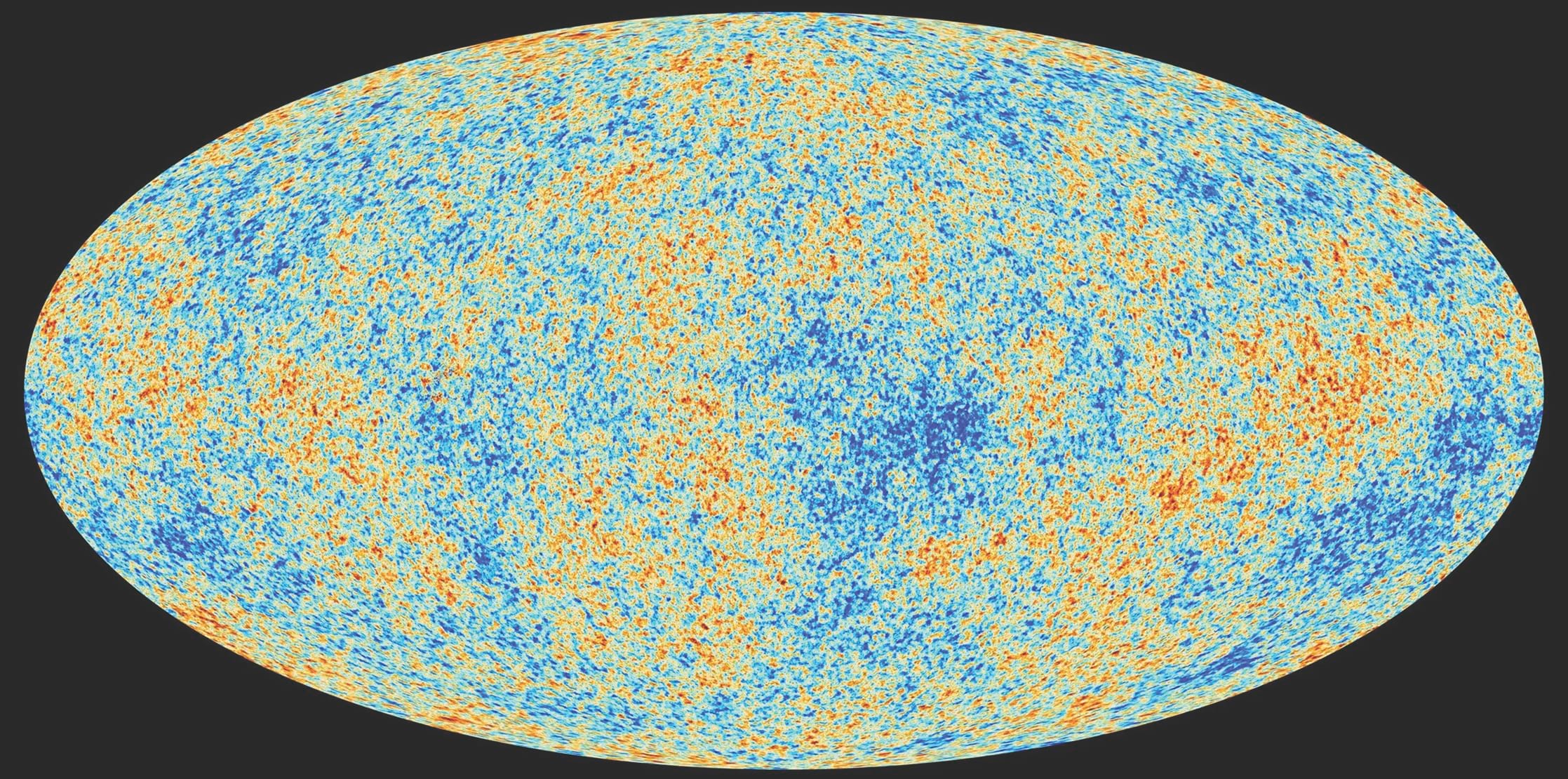
Cosmic microwave radiation left over from the Big Bang, as seen by the Planck spacecraft – NASA
Additionally, access to data is another challenge. Not all the datasets are publicly available. Either they have not been released, or they are only partially available in research papers. ‘What you can do is refer to a previous data set, and then you try to link your results to the newer results. This is yet another challenge as you have to interpret old data from the point of view that is currently being explored by new datasets,’ Mifsud says.
And the data analysis is lengthy. Results are not delivered by the machine instantly; sometimes researchers have to wait for weeks to see the results of their calculations. Should they need to rerun the analysis, it can put them back by several weeks.
One Giant Leap for Machine (Learning)
The project is still ongoing. The core interest of IntelliVerse is getting a better model of the universe. The researchers have developed a number of different codes that take advantage of novel techniques in machine learning, which they use to develop tools to interpret cosmological data in the most model- or physics-independent way possible. The codes, all based on foundations of machine learning techniques, are used in different scenarios to expose possible indications of new physics through the Hubble Constant Crisis.
‘We hope to convince the community that ours is a serious approach to addressing the age of the universe. We have some good models and explanations coming out in the near future, in some interesting papers that we hope may be able to ease the Hubble Constant Tension,’ Said says.
Machine learning can help remove elements of underlying physics from certain data sets. This allows the researchers to prove actual physics theories against pure or physics-free data. Machine learning can thus help build better pipelines by which the testing of different physics models becomes possible.
‘We are also interested in developing machine learning tools that can take several physics models and decide which of these are better models that describe the observational data. When the space of models is enlarged to a large number of physics models, we tend to have a situation that would not be possible to scan manually,’ Said adds.
Machine learning has already shown promise in many aspects of science, and the IntelliVerse project hopes to transfer that disruption to cosmology. ‘There is always a lot of inertia in research, but once it starts, it snowballs into an avalanche. That is exactly what we are hoping for,’ Said concludes.
Comments are closed for this article!